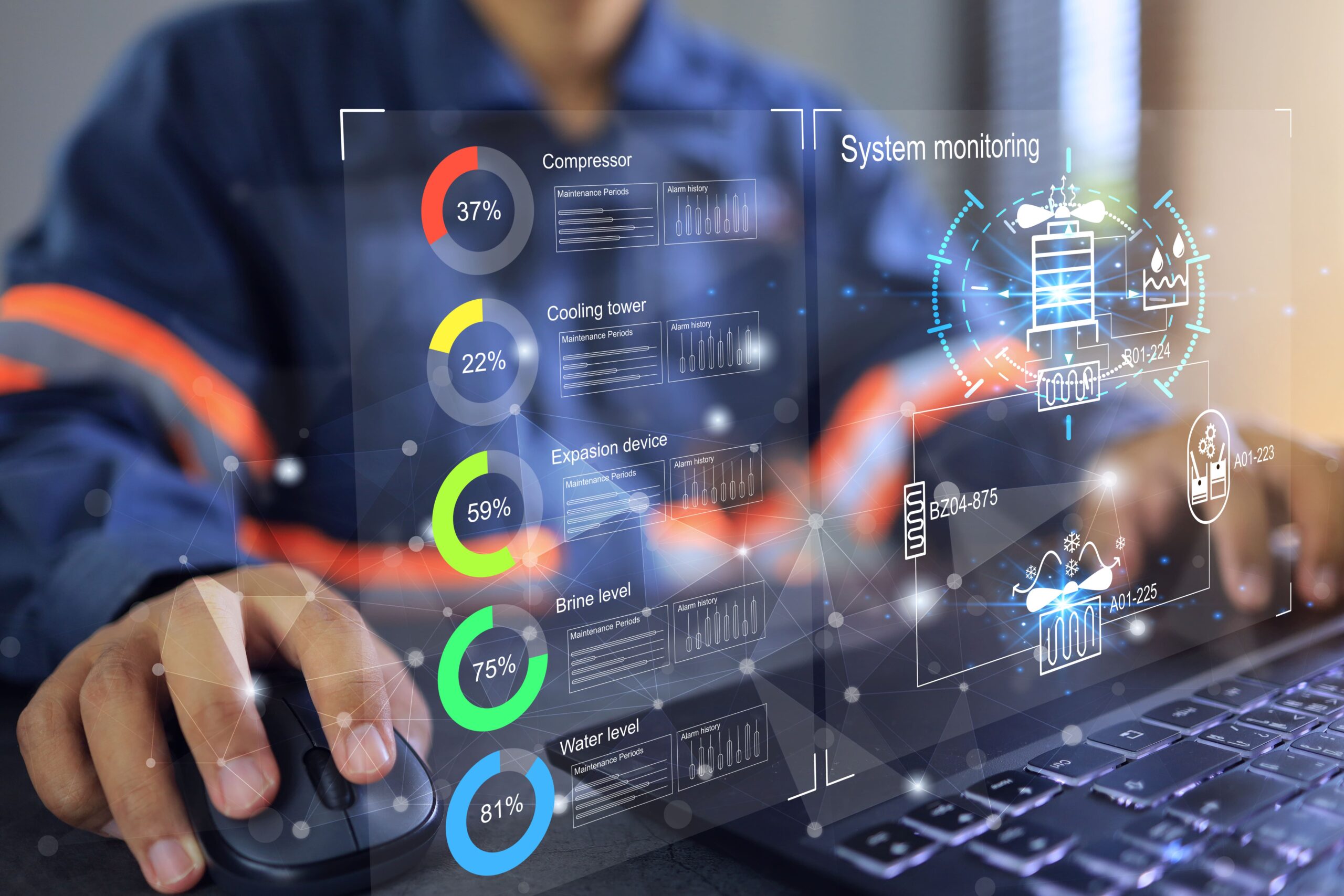
DCARBO Project
Real-time monitoring
Decarbonising industry requires better control and refined knowledge of CO₂ emissions from production systems. Installing sensors at strategic positions and managing production data in real time provides relevant information to enable dynamic analysis of CO₂ emissions with greater accuracy than current asynchronous approaches.
The challenge is to define, for different types of production systems, the appropriate structure for relevant data acquisition and collection as well as for the associated decision-making instruments for decarbonisation.
The aim is to propose suitable artificial intelligence models and algorithms for each decision-making level, to experiment with and compare different models and algorithms, and to establish a methodology for implementing new approaches in industrial contexts. The originality of this research lies in the coupling of different types of AI and their application to the real-time monitoring and control of industrial processes, in order to promote the effective decarbonisation of industries.
The main difficulties of this project will be linked to the challenge of finding the right level of information/instrumentation for the processes and the complexity of managing simulations for short-term and real-time decision-making.
Five-year term – Budget of €1.68M
Implementing a methodology for monitoring indicators linked to one or more production units, reflecting the environmental impact of this unit, based on its various components: energy consumption, resources and emissions linked to water, air and soil.
Three types of process will be concerned: discrete processes (manufacturing industries, microelectronics), continuous processes (cement works, local distribution players for heat networks) and computer and storage centres for digital industries. It will be important to identify the differences between these types of processes and the impact on the method to be developed. A link will be made with the targeted dynamic LCA project.
TRL 4 at the end of the project.
Tasks undertaken : development of an approach to identify and monitor the main contributors to greenhouse gases in production systems – development of digital tools for industrial decarbonisation (data mining algorithms for data pre-processing and process understanding – principal component analysis PCA – Locally linear embedding LLE, MSPC Multivariate Statistical Process control, hybrid AI for data drift detection and recommendation actions – machine learning algorithm such as deep neural network DNN, decision tree DT association rules learning algorithm – development of optimisation approaches to understand decarbonisation in an efficient way, explore carbon emissions within digital industry), proposal of a framework for the management of industrial systems aimed at decarbonisation).
Synergy
LCA, CEI sensors (Corporate engagement and innovation)
The consortium
IFPEN, CNRS + G-SCOP, Grenoble
Coordination
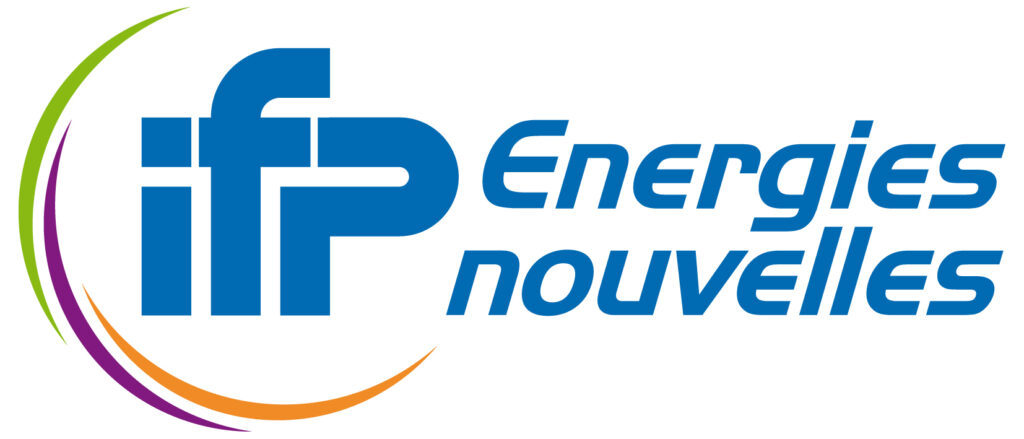
More projects
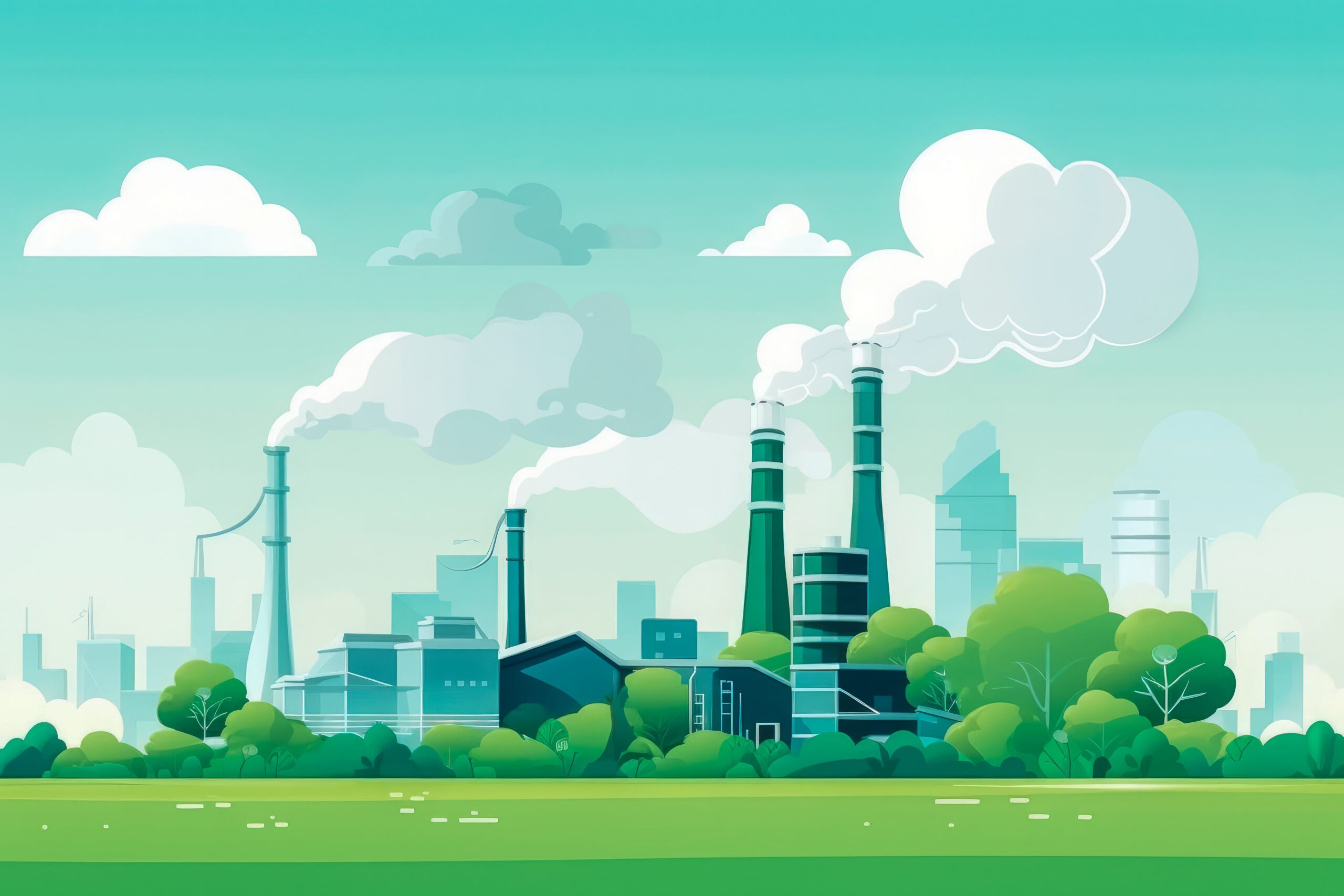
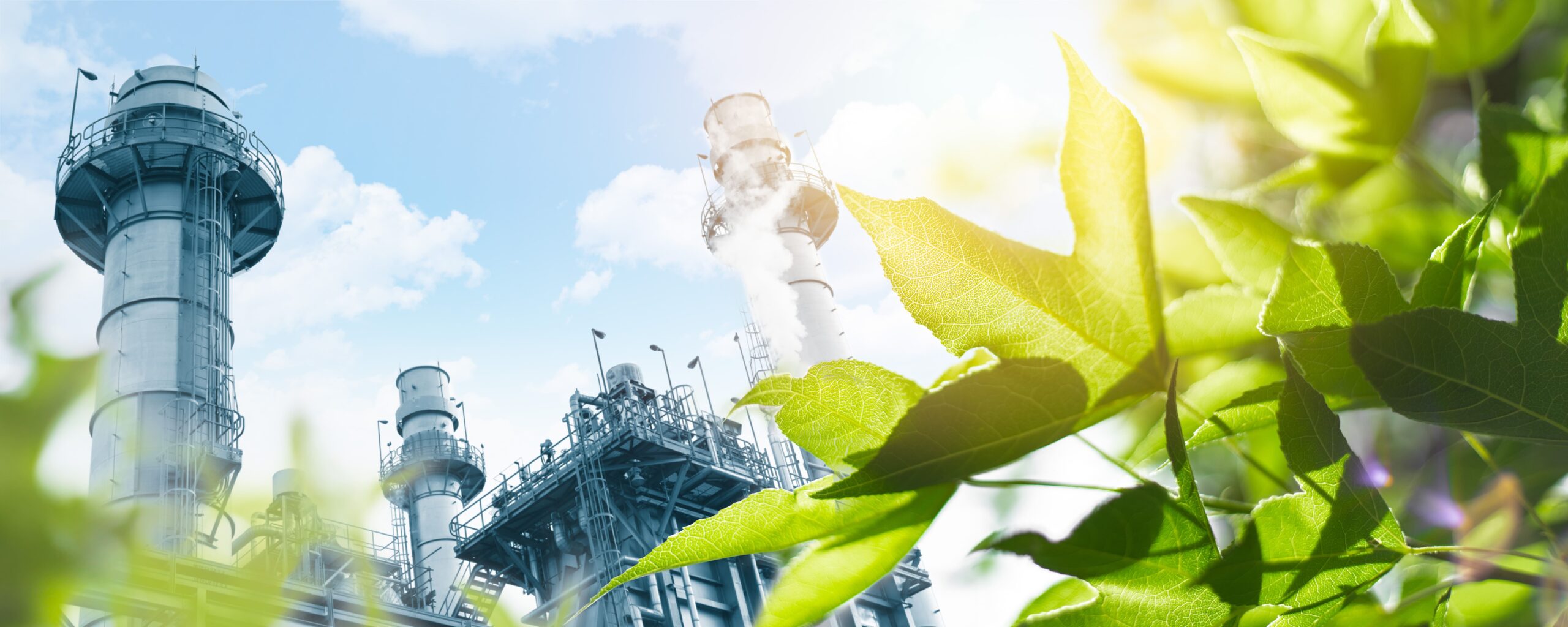